Observations¶
For all environments, several types of observations can be used. They are defined in the
observation
module.
Each environment comes with a default observation, which can be changed or customised using
environment configurations. For instance,
import gym
import highway_env
env = gym.make('highway-v0')
env.configure({
"observation": {
"type": "OccupancyGrid",
"vehicles_count": 15,
"features": ["presence", "x", "y", "vx", "vy", "cos_h", "sin_h"],
"features_range": {
"x": [-100, 100],
"y": [-100, 100],
"vx": [-20, 20],
"vy": [-20, 20]
},
"grid_size": [[-27.5, 27.5], [-27.5, 27.5]],
"grid_step": [5, 5],
"absolute": False
}
})
env.reset()
Note
The "type"
field in the observation configuration takes values defined in
observation_factory()
(see source)
Kinematics¶
The KinematicObservation
is a \(V\times F\) array that describes a
list of \(V\) nearby vehicles by a set of features of size \(F\), listed in the "features"
configuration field.
For instance:
Vehicle |
\(x\) |
\(y\) |
\(v_x\) |
\(v_y\) |
---|---|---|---|---|
ego-vehicle |
5.0 |
4.0 |
15.0 |
0 |
vehicle 1 |
-10.0 |
4.0 |
12.0 |
0 |
vehicle 2 |
13.0 |
8.0 |
13.5 |
0 |
… |
… |
… |
… |
… |
vehicle V |
22.2 |
10.5 |
18.0 |
0.5 |
Note
The ego-vehicle is always described in the first row
If configured with normalized=True
(default), the observation is normalized within a fixed range, which gives for
the range [100, 100, 20, 20]:
Vehicle |
\(x\) |
\(y\) |
\(v_x\) |
\(v_y\) |
---|---|---|---|---|
ego-vehicle |
0.05 |
0.04 |
0.75 |
0 |
vehicle 1 |
-0.1 |
0.04 |
0.6 |
0 |
vehicle 2 |
0.13 |
0.08 |
0.675 |
0 |
… |
… |
… |
… |
… |
vehicle V |
0.222 |
0.105 |
0.9 |
0.025 |
If configured with absolute=False
, the coordinates are relative to the ego-vehicle, except for the ego-vehicle
which stays absolute.
Vehicle |
\(x\) |
\(y\) |
\(v_x\) |
\(v_y\) |
---|---|---|---|---|
ego-vehicle |
0.05 |
0.04 |
0.75 |
0 |
vehicle 1 |
-0.15 |
0 |
-0.15 |
0 |
vehicle 2 |
0.08 |
0.04 |
-0.075 |
0 |
… |
… |
… |
… |
… |
vehicle V |
0.172 |
0.065 |
0.15 |
0.025 |
Note
The number \(V\) of vehicles is constant and configured by the vehicles_count
field, so that the
observation has a fixed size. If fewer vehicles than vehicles_count
are observed, the last rows are placeholders
filled with zeros. The presence
feature can be used to detect such cases, since it is set to 1 for any observed
vehicle and 0 for placeholders.
Example configuration¶
"observation": {
"type": "Kinematics",
"vehicles_count": 15,
"features": ["presence", "x", "y", "vx", "vy", "cos_h", "sin_h"],
"features_range": {
"x": [-100, 100],
"y": [-100, 100],
"vx": [-20, 20],
"vy": [-20, 20]
},
"absolute": False,
"order": "sorted"
}
Grayscale Image¶
The GrayscaleObservation
is a \(W\times H\) grayscale image of the scene, where \(W,H\) are set with the observation_shape
parameter.
The RGB to grayscale conversion is a weighted sum, configured by the weights
parameter. Several images can be stacked with the stack_size
parameter, as is customary with image observations.
The following images illustrate the stacking process for the first four observations, using the example configuration below.
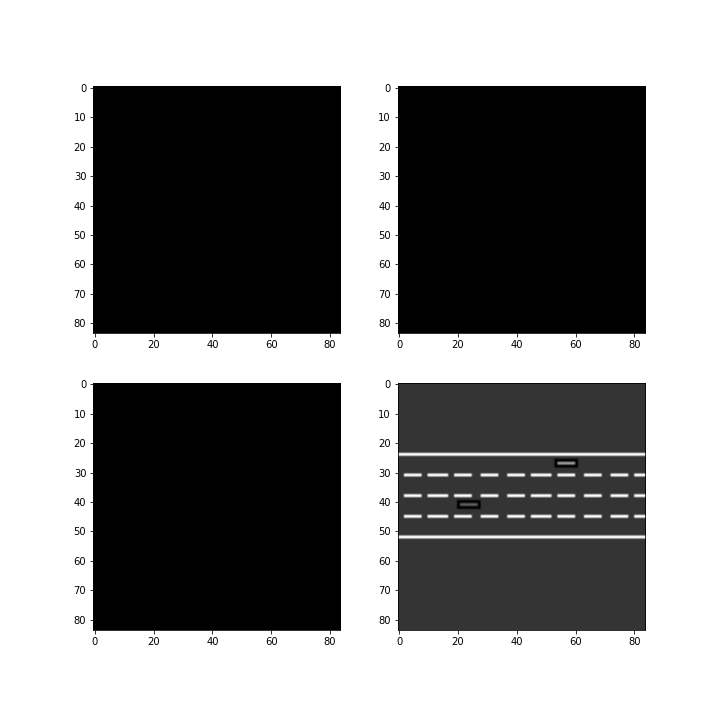
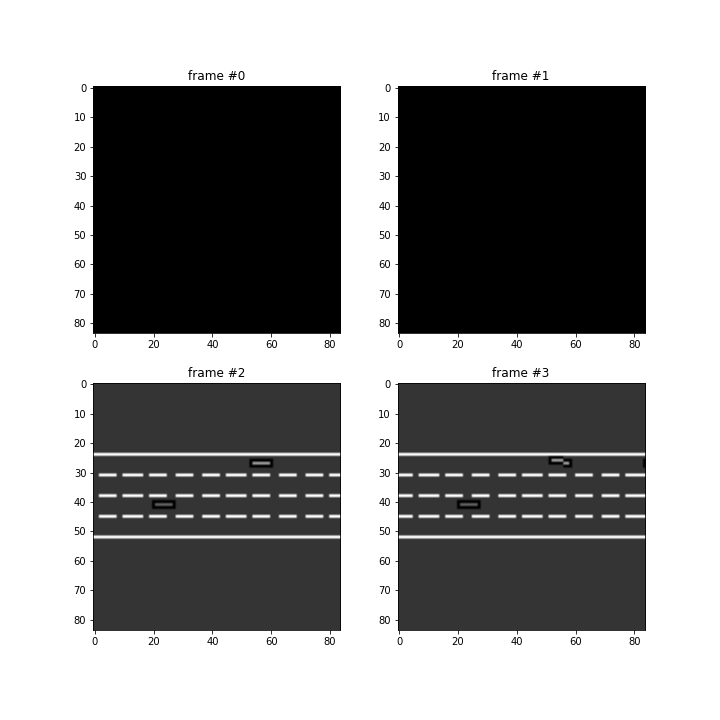
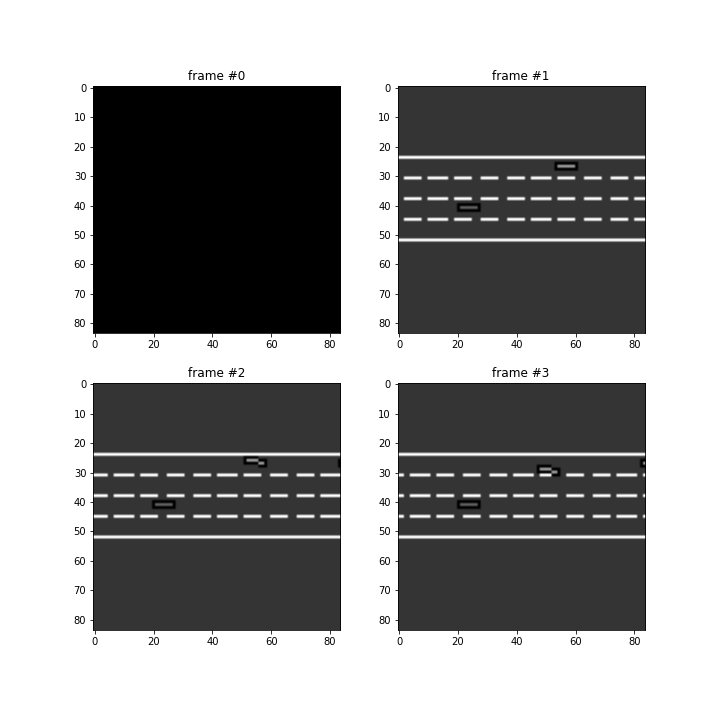
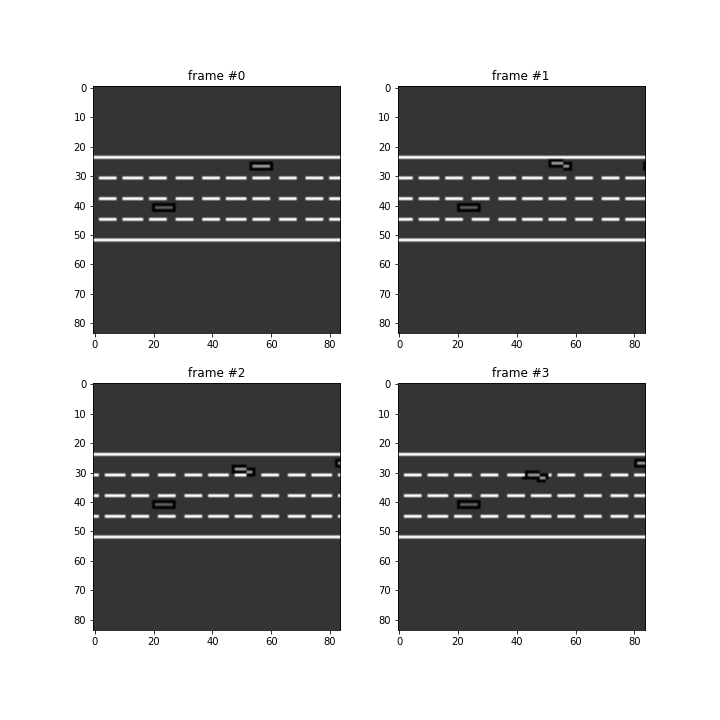
Warning
The screen_height
and screen_width
environment configurations should match the expected observation_shape
.
Warning
This observation type required pygame rendering, which may be problematic when run on server without display. In this case, the call to pygame.display.set_mode()
raises an exception, which can be avoided by setting the environment configuration offscreen_rendering
to True
.
Example configuration¶
screen_width, screen_height = 84, 84
config = {
"offscreen_rendering": True,
"observation": {
"type": "GrayscaleObservation",
"weights": [0.2989, 0.5870, 0.1140], # weights for RGB conversion
"stack_size": 4,
"observation_shape": (screen_width, screen_height)
},
"screen_width": screen_width,
"screen_height": screen_height,
"scaling": 1.75,
"policy_frequency": 2
}
env.configure(config)
Occupancy grid¶
The OccupancyGridObservation
is a \(W\times H\times F\) array,
that represents a grid of shape \(W\times H\) discretising the space \((X,Y)\) around the ego-vehicle in
uniform rectangle cells. Each cell is described by \(F\) features, listed in the "features"
configuration field.
The grid size and resolution is defined by the grid_size
and grid_steps
configuration fields.
For instance, the channel corresponding to the presence
feature may look like this:
0 |
0 |
0 |
0 |
0 |
0 |
0 |
1 |
0 |
0 |
0 |
0 |
0 |
0 |
1 |
0 |
0 |
0 |
0 |
0 |
0 |
0 |
0 |
0 |
0 |
The corresponding \(v_x\) feature may look like this:
0 |
0 |
0 |
0 |
0 |
0 |
0 |
0 |
0 |
0 |
0 |
0 |
0 |
0 |
-0.1 |
0 |
0 |
0 |
0 |
0 |
0 |
0 |
0 |
0 |
0 |
Example configuration¶
"observation": {
"type": "OccupancyGrid",
"vehicles_count": 15,
"features": ["presence", "x", "y", "vx", "vy", "cos_h", "sin_h"],
"features_range": {
"x": [-100, 100],
"y": [-100, 100],
"vx": [-20, 20],
"vy": [-20, 20]
},
"grid_size": [[-27.5, 27.5], [-27.5, 27.5]],
"grid_step": [5, 5],
"absolute": False
}
Time to collision¶
The TimeToCollisionObservation
is a \(V\times L\times H\) array, that represents the predicted time-to-collision of observed vehicles on the same road as the ego-vehicle.
These predictions are performed for \(V\) different values of the ego-vehicle speed, \(L\) lanes on the road around the current lane, and represented as one-hot encodings over \(H\) discretised time values (bins), with 1s steps.
For instance, consider a vehicle at 25m on the right-lane of the ego-vehicle and driving at 15 m/s. Using \(V=3,\, L = 3\,H = 10\), with ego-speed of {\(15\) m/s, \(20\) m/s and \(25\) m/s}, the predicted time-to-collisions are \(\infty,\,5s,\,2.5s\) and the corresponding observation is
0 |
0 |
0 |
0 |
0 |
0 |
0 |
0 |
0 |
0 |
0 |
0 |
0 |
0 |
0 |
0 |
0 |
0 |
0 |
0 |
0 |
0 |
0 |
0 |
0 |
0 |
0 |
0 |
0 |
0 |
0 |
0 |
0 |
0 |
0 |
0 |
0 |
0 |
0 |
0 |
0 |
0 |
0 |
0 |
0 |
0 |
0 |
0 |
0 |
0 |
0 |
0 |
0 |
0 |
1 |
0 |
0 |
0 |
0 |
0 |
0 |
0 |
0 |
0 |
0 |
0 |
0 |
0 |
0 |
0 |
0 |
0 |
0 |
0 |
0 |
0 |
0 |
0 |
0 |
0 |
0 |
0 |
1 |
0 |
0 |
0 |
0 |
0 |
0 |
0 |
Example configuration¶
"observation": {
"type": "TimeToCollision"
"horizon": 10
},
API¶
-
class
highway_env.envs.common.observation.
ObservationType
[source]¶ -
-
__dict__
= mappingproxy({'__module__': 'highway_env.envs.common.observation', 'space': <function ObservationType.space>, 'observe': <function ObservationType.observe>, '__dict__': <attribute '__dict__' of 'ObservationType' objects>, '__weakref__': <attribute '__weakref__' of 'ObservationType' objects>, '__doc__': None})¶
-
__module__
= 'highway_env.envs.common.observation'¶
-
__weakref__
¶ list of weak references to the object (if defined)
-
-
class
highway_env.envs.common.observation.
GrayscaleObservation
(env: AbstractEnv, config: dict)[source]¶ An observation class that collects directly what the simulator renders
Also stacks the collected frames as in the nature DQN. Specific keys are expected in the configuration dictionary passed.
- Example of observation dictionary in the environment config:
- observation”: {
“type”: “GrayscaleObservation”, “weights”: [0.2989, 0.5870, 0.1140], #weights for RGB conversion, “stack_size”: 4, “observation_shape”: (84, 84)
}
Also, the screen_height and screen_width of the environment should match the expected observation_shape.
-
__init__
(env: AbstractEnv, config: dict) → None[source]¶ Initialize self. See help(type(self)) for accurate signature.
-
__module__
= 'highway_env.envs.common.observation'¶
-
class
highway_env.envs.common.observation.
TimeToCollisionObservation
(env: AbstractEnv, horizon: int = 10, **kwargs: dict)[source]¶ -
__init__
(env: AbstractEnv, horizon: int = 10, **kwargs: dict) → None[source]¶ Initialize self. See help(type(self)) for accurate signature.
-
__module__
= 'highway_env.envs.common.observation'¶
-
-
class
highway_env.envs.common.observation.
KinematicObservation
(env: AbstractEnv, features: List[str] = None, vehicles_count: int = 5, features_range: Dict[str, List[float]] = None, absolute: bool = False, order: str = 'sorted', normalize: bool = True, clip: bool = True, see_behind: bool = False, observe_intentions: bool = False, **kwargs: dict)[source]¶ Observe the kinematics of nearby vehicles.
-
FEATURES
: List[str] = ['presence', 'x', 'y', 'vx', 'vy']¶
-
__init__
(env: AbstractEnv, features: List[str] = None, vehicles_count: int = 5, features_range: Dict[str, List[float]] = None, absolute: bool = False, order: str = 'sorted', normalize: bool = True, clip: bool = True, see_behind: bool = False, observe_intentions: bool = False, **kwargs: dict) → None[source]¶ - Parameters
env – The environment to observe
features – Names of features used in the observation
vehicles_count – Number of observed vehicles
absolute – Use absolute coordinates
order – Order of observed vehicles. Values: sorted, shuffled
normalize – Should the observation be normalized
clip – Should the value be clipped in the desired range
see_behind – Should the observation contains the vehicles behind
observe_intentions – Observe the destinations of other vehicles
-
normalize_obs
(df: pandas.core.frame.DataFrame) → pandas.core.frame.DataFrame[source]¶ Normalize the observation values.
For now, assume that the road is straight along the x axis. :param Dataframe df: observation data
-
__annotations__
= {'FEATURES': typing.List[str]}¶
-
__module__
= 'highway_env.envs.common.observation'¶
-
-
class
highway_env.envs.common.observation.
OccupancyGridObservation
(env: AbstractEnv, features: Optional[List[str]] = None, grid_size: Optional[List[List[float]]] = None, grid_step: Optional[List[int]] = None, features_range: Dict[str, List[float]] = None, absolute: bool = False, **kwargs: dict)[source]¶ Observe an occupancy grid of nearby vehicles.
-
FEATURES
: List[str] = ['presence', 'vx', 'vy']¶
-
GRID_SIZE
: List[List[float]] = [[-27.5, 27.5], [-27.5, 27.5]]¶
-
GRID_STEP
: List[int] = [5, 5]¶
-
__init__
(env: AbstractEnv, features: Optional[List[str]] = None, grid_size: Optional[List[List[float]]] = None, grid_step: Optional[List[int]] = None, features_range: Dict[str, List[float]] = None, absolute: bool = False, **kwargs: dict) → None[source]¶ - Parameters
env – The environment to observe
features – Names of features used in the observation
vehicles_count – Number of observed vehicles
-
normalize
(df: pandas.core.frame.DataFrame) → pandas.core.frame.DataFrame[source]¶ Normalize the observation values.
For now, assume that the road is straight along the x axis. :param Dataframe df: observation data
-
__annotations__
= {'FEATURES': typing.List[str], 'GRID_SIZE': typing.List[typing.List[float]], 'GRID_STEP': typing.List[int]}¶
-
__module__
= 'highway_env.envs.common.observation'¶
-
-
class
highway_env.envs.common.observation.
KinematicsGoalObservation
(env: AbstractEnv, scales: List[float], **kwargs: dict)[source]¶ -
__init__
(env: AbstractEnv, scales: List[float], **kwargs: dict) → None[source]¶ - Parameters
env – The environment to observe
features – Names of features used in the observation
vehicles_count – Number of observed vehicles
absolute – Use absolute coordinates
order – Order of observed vehicles. Values: sorted, shuffled
normalize – Should the observation be normalized
clip – Should the value be clipped in the desired range
see_behind – Should the observation contains the vehicles behind
observe_intentions – Observe the destinations of other vehicles
-
__module__
= 'highway_env.envs.common.observation'¶
-
-
class
highway_env.envs.common.observation.
AttributesObservation
(env: AbstractEnv, attributes: List[str], **kwargs: dict)[source]¶ -
__init__
(env: AbstractEnv, attributes: List[str], **kwargs: dict) → None[source]¶ Initialize self. See help(type(self)) for accurate signature.
-
__module__
= 'highway_env.envs.common.observation'¶
-